Thanks for subscribing to the free version of RMN. Here’s what paid subscribers read this week:
Texas Hires Willis For Rate, Model Review After Pushback
All Pandemic Modeling Is Local
New Flood Model Floods Media Coverage
Consider subscribing to Risk Market News
Earlier this week researchers in Boston published their insights into modeling the current COVID-19 pandemic which says that national forecasting models often brake down regionally because there are few if any local variables considered.
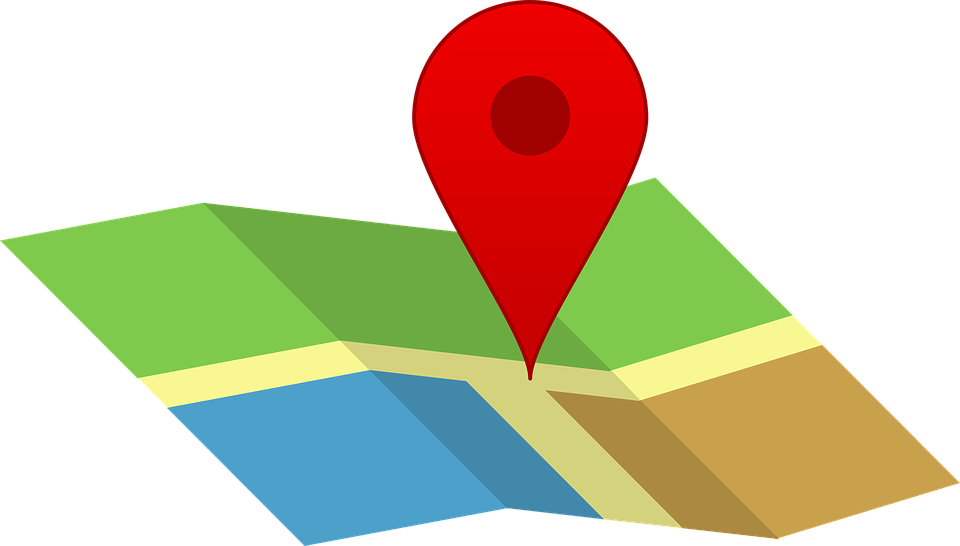
RMN spoke with two authors of the study: Dr. Jennifer P. Stevens, Director of the Center for Healthcare Delivery Science and an Assistant Professor at Harvard Medical School and Dr. Steven Horng, Clinical Lead for Machine Learning at the Center for Healthcare Delivery Science and instructor in Emergency Medicine at Harvard Medical School.
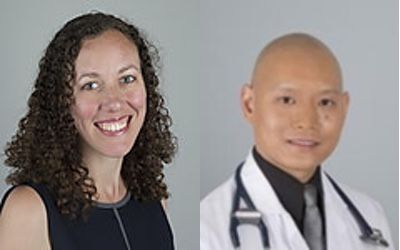
Risk Market News: The thesis of your project seems to be that the broader COVID-19 models aren't capturing the information that local community health providers would find valuable. Are there deficiencies in national models and do you still use them in any capacity?
Dr. Jennifer Stevens: At the beginning, in March, both our model and national models were all facing the same challenge – trying to develop forecasting tools about a virus that was largely unknown.
National models are trying to produce a product for a different audience than what an individual hospital needs.
A local hospital needs to make decisions about when to close elective procedures, which is a major financial consideration for an institution. They need to ask how to use personal protective equipment (PPE) in a way that protects patients and providers, and how to use PPE when you start having elective procedures again. The individual needs of an institution are different than the output produced by a national model.
Finally, when it comes to predicting things at a hospital level, we needed to understand what the hospital itself was doing. There were individual decisions that our hospital was making, for example, the number of COVID-19 positive patients in the emergency room that were going to be admitted to the hospital floors. Or the timing of invasive mechanical ventilation for patients with respiratory distress with COVID-19 that were specific to our hospital, and also changed over time as we progressed through the epidemic. Those are other things that lead to different outputs at a hospital level than would be different at a national level.
Dr. Steven Horng: One thing that I might point out is that an advantage of a national model is you have a much larger sample size. Because we think of epidemics as stochastic processes, the more observations that you have, the better one can train a model. The problem with that is if you look at the United States, it isn't homogeneous in how the virus has spread. Now, even if you look at it at the state level, within each state it's not homogeneous.
That's what a hyperlocal model really gets you, the ability to look at a very small segment that's more homogeneous and is able to give you predictions for that one area.
One of the things that we've done is used a machine learning technique called multi-task learning. So even though the number of observations for these hyperlocal areas are small, we're able to take advantage of learning from all these areas simultaneously, exploiting commonalities across areas, thereby taking advantage of large numbers across areas, while still learning hyperlocal models.
If you were an insurance company and you were trying to determine the risk for a flood, you wouldn’t draw a flood plain map with a granularity at the entire state-level, you would do it for a small geographic area, in order to be actionable. And that's similar to how the modeling for COVID-19 has to be, because sure it's important from the government perspective of what the entire state is doing, but for an individual homeowner or for an individual hospital, what you care about is what's going to happen in your home or at your hospital.
RMN: What are the challenges of getting that data needed at the local level?
Stevens: Our data came from essentially three major sources.
One was learning about the infection’s characteristics. Those are parameters we can learn from the research community at large, which subsequently changed over time.
The second major pile of data comes from a cell phone transmission and mobility data. There have been a number of companies that have come forward to make some of those data more publicly available.
We used that two data sets to understand some of these hyperlocal shifts in population that Dr. Horng was talking about. For example, Boston is a college town so early on when the governor shut colleges down very specific neighborhoods where the students live emptied out. If you use a traditional epidemiological framework of an SIR Model (Susceptible, Infected and Recovered), it presumes a homogenous mixing population. That's clearly not the case anymore. And if you just looked at census data for Boston alone, you would be wrong in the number of people moving susceptible to the infection.
The third source of data which we used were originally sourced from our own institution. Beth Israel Deaconess Medical Center is a member of a network of hospitals, so we are able to be able to learn the different patterns of behavior across the hospitals. That data we use predominantly census data, and is fundamentally publicly available through the Massachusetts department of public health.
One of the premises of how we try to design this model is the idea we should have more generalizable learnings from this. If we could show the benefit of a hyperlocal model for our institution, you would be able to export that in some manner to another institution.
I think the hypothesis that you can take these publicly available data sources or semi publicly available data sources with local knowledge to create a more useful and a predictive model is the premise that we built this on.
RMN: Boston based hospital systems, centered among several world-class universities, has access to a lot of resources and a lot of data. Would this model or would this process work out at a regional hospital in Midwest where they don't have that same access?
Stevens: I think there is a larger question of does an institution essentially invest in people who do this kind of research to begin with. So as a modest size hospital may or may not do that, but you could imagine that maybe there is a greater market for the sort of member of the C suite or a person who reports to the C suite.
So our institution is able to do that on a different scale because you're right, we're a large academic health system. But fundamentally, it's a decision by the hospital to support the flexibility, for us to come in and assess the analytic needs of a problem like the pandemic, and then use research tools like epidemiology and machine learning to solve them.
Horng: The way we're approaching epidemiological modelling is a hybrid of traditional epidemiological models and modern machine learning methods. We’re specifically using multi-task learning what allows us to share parameters across different hyperlocal models in order to decrease the sample size that's required to train a good model.
For example, if you're from a really small hospital, you may not have enough of a sample size to train a model well. The way we've constructed the model allows us take advantage of all the hospitals you have and -- big and small -- and learn shared parameters that are intrinsic to the virus, that are identical across models, regardless of where you are. Then, there are some local parameters that are more locally driven, based on the hospital culture and hospital resources.
For example, the incubation period of the virus is something that's intrinsic to the virus. The number of days that your infectious and the intrinsic doubling rate are all intrinsic to our human physiology and the virus, as long as the virus doesn’t mutate. Those are parameters that we can learn and share across the different models for each locale.
Lasatly, we specifically chose census data because it's easily accessible for every hospital. By doing so, we can now scale this to any hospital that chooses to participate. And, in fact, the more hospitals that we recruit, the better model that we build for everyone.
RMN: How do you employ machine learning in the model?
Horng: In traditional epidemiological models such as S-I-R models, one has to know the parameters that specify the model exactly. You get those parameters from prior studies and from the published evidence.
Now, what we know is that because it's so early in the days of the pandemic and because everyone's experience has been so different, we really don't know what the true values of the parameters are. Those parameters really change a lot of how traditional S-I-R models project.
What we do instead is take a hybrid approach, using machine learning to learn the parameters for a S-I-R like model. A traditional S-I-R model using ordinary differential equations, and assumes a closed system where no one enters or leaves the system. The parameters of the model are also fixed over time. We instead use a more general Markov model, relaxing these assumptions, allowing parameters such as population, social distancing, etc. to change over time.
We use multi-task learning to jointly train each of these hyperlocal models simultaneously, sharing parameters that are intrinsic to the virus, while allowing for local parameters that are specific to each area, through a joint objective function, estimating those parameters using a non-convex Bayesian optimization technique called tree-structured parzen estimators. Local parameters would be things like the population, amount of mobility, compliance with social distancing recommendations, etc. over time.
One question is because we're using incidence data and not prevalence data is whether this model is identifiable or not. And the way we mitigate the chances of learning the wrong model is by using multi-task learning, where we have a joint objective function across all these different models, where we do both hard parameter sharing and soft parameter sharing.
All this to be said, that's what allows us to learn across all these different locales, but still have the statistical power of all of them together. So that's a technique, especially in the last few years, that has been instrumental to machine learning world to learn from small samples.
RMN: So how does this all fit into how you validate the model?
Stevens: The way we validate is run the model under a certain date and see how it performs in the next seven to 14 to 21 days.
The way we evaluate is to look at our calibration and discrimination at a given time point and then going forward. Fundamentally, that’s because that's who the customer is. The customers are institutions that are interested in “what do I need to know for the next seven days? What do I need to know for the next 14 days? How confident are you about that?”
Fundamentally, we also function as an alert system, like a weather prediction service. We alert when something bad is coming possibly in the distant future or in a couple of months, but all of that reflects the difficulty of the other aspects of the changing environment. So, what is the flu season going to be like this year? I don't know, hard to say. That's definitely going to play a factor this fall, and we just have the flexibility in our modeling to be able to incorporate those early learnings for longer term projections.
RMN: Should there be more focus on regional and even hyperlocal models throughout the US?
Stevens: I think that there's certainly a clear health and economic benefits to considering the hyperlocal approach in health in terms of understanding patterns of behavior as these different waves of infection wash over different regions. From an economic standpoint, what are the consequences of some of those and what are the true consequences of different economic decisions? I think the pattern of either people building these national models or building local models is a reflection of the fact that we are trying to estimate things that potentially we should be learning from other more classical public health strategies. So, a broader testing patterns would tell us more information about who was infected, where they were infected and how they're moving.
My take would be that there have been many models because we are trying to mathematically understand the behavior of this virus and behavior of people with this virus, because that is a stand in or like real, more gumshoe epidemiology that might've existed in a different time and a different place. I am all in support of greater federal support for this type of research because I think there's benefit to that. But modeling in itself strikes me as a solution to a problem that's created by not having the same knowledge about the virus.
RMN: What's your hope for next steps for this project and where would you like to see it go?
Stevens: I would like to see this kind of tool be more available to individual hospitals systems. I would like to see our tool be both trained on and expanded to institutions that may not have these sorts of resources.
The more prepared hospital systems are the more lives are saved and the more health healthcare professionals have the equipment that they need. I would like to see what we learn about the consequences of different public health strategies and behaviors and shifts in population to translate into helpful guidance in those areas. We do have a broad national experiment right now going on of different types of reopening and different types of public health strategies.
And we are able to take advantage of some of the data that we have to study that locally so that we can understand what to do for our Massachusetts residents, but that's only helping the people that we care for.
And then fundamentally, I would like to see support for this type of approach for health care operations more generally. Sometimes you need different research strategies to answer questions for health care leaders. And we were fortunate to be available to do that. I'd like to see that be more available because I feel like that helps hospitals run better.
Horng: I completely agree with Jen's thoughts. We are in a very privileged position here in being surrounded by very smart people who work on this type of thing, and an environment which supports this type of work. And because of that, we have analytical capabilities that are second to none. And we're able to produce models that are really quite innovative. And so, my hope for this project is that we can deliver the same type of actionable decision support to any hospital so that decision makers can make data-driven decisions for any hospital system regardless of its size.
Hopefully, we can become a learning health system, so that we aren't just learning from our own experiences, but we can learn from others as well. So, for example, certain states have opened up earlier and we've learned from that experience of what it means to increase transmissibility because of increased mobility. And it's not just mobility alone, it's not just that you go out, it’s where you go and what you do when you get there. And those are experiences that we can learn from so that we don’t repeat those same experiences.